[ad_1]
A team of researchers from British universities has trained a deep learning model that can steal data from keyboard keystrokes recorded using a microphone with an accuracy of 95%.
When Zoom was used for training the sound classification algorithm, the prediction accuracy dropped to 93%, which is still dangerously high, and a record for that medium.
Such an attack severely affects the target’s data security, as it could leak people’s passwords, discussions, messages, or other sensitive information to malicious third parties.
Moreover, contrary to other side-channel attacks that require special conditions and are subject to data rate and distance limitations, acoustic attacks have become much simpler due to the abundance of microphone-bearing devices that can achieve high-quality audio captures.
This, combined with the rapid advancements in machine learning, makes sound-based side-channel attacks feasible and a lot more dangerous than previously anticipated.
Listening to keystrokes
The first step of the attack is to record keystrokes on the target’s keyboard, as that data is required for training the prediction algorithm. This can be achieved via a nearby microphone or the target’s phone that might have been infected by malware that has access to its microphone.
Alternatively, keystrokes can be recorded through a Zoom call where a rogue meeting participant makes correlations between messages typed by the target and their sound recording.
The researchers gathered training data by pressing 36 keys on a modern MacBook Pro 25 times each and recording the sound produced by each press.
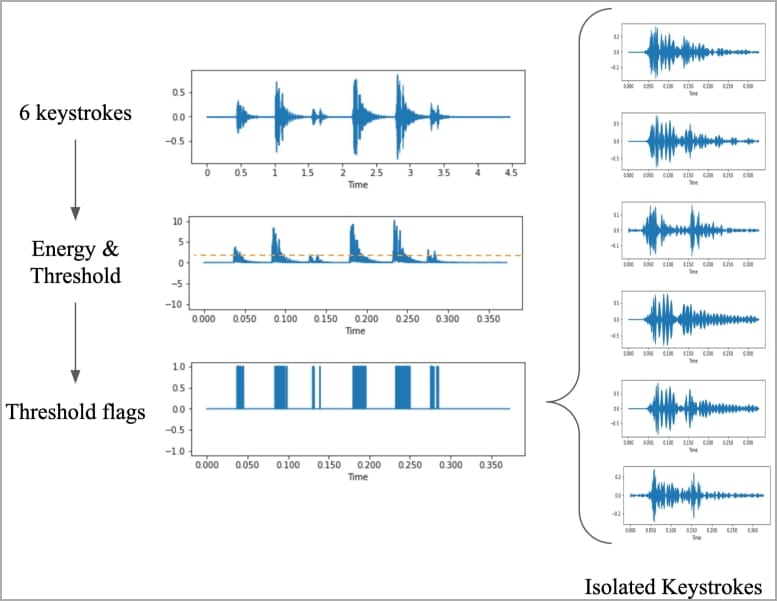
Then, they produced waveforms and spectrograms from the recordings that visualize identifiable differences for each key and performed specific data processing steps to augment the signals that can be used for identifying keystrokes.
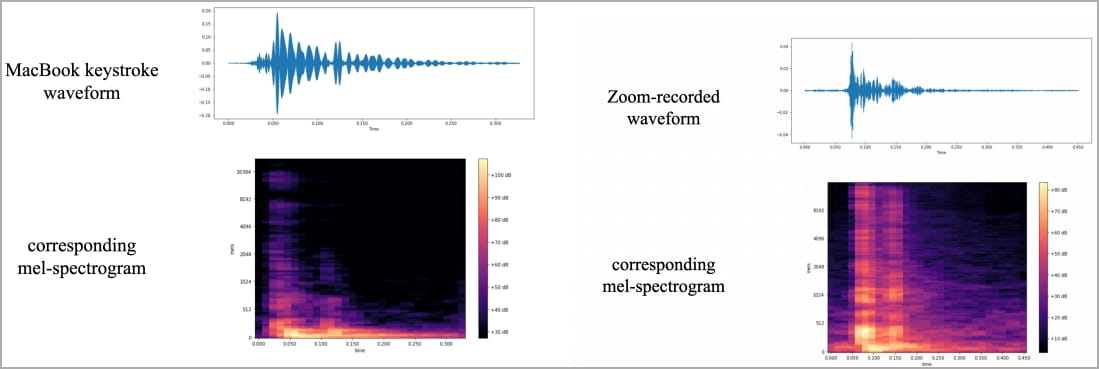
The spectrogram images were used to train ‘CoAtNet,’ which is an image classifier, while the process required some experimentation with epoch, learning rate, and data splitting parameters until the best prediction accuracy results could be achieved.
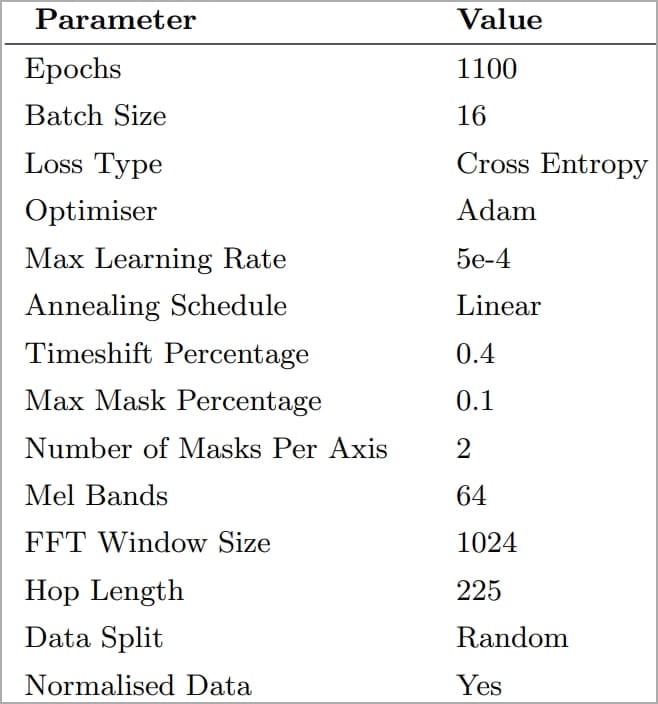
In their experiments, the researchers used the same laptop, whose keyboard has been used in all Apple laptops for the past two years, an iPhone 13 mini placed 17cm away from the target, and Zoom.
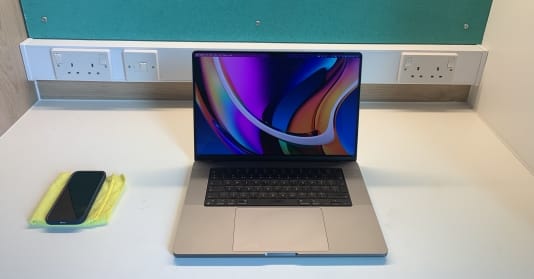
The CoANet classifier achieved 95% accuracy from the smartphone recordings and 93% from those captured through Zoom. Skype produced a lower but still usable 91.7% accuracy.
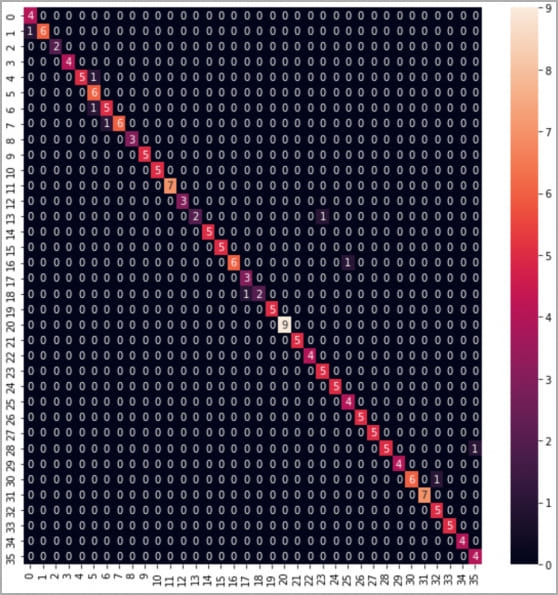
Possible mitigations
For users who are overly worried about acoustic side-channel attacks, the paper suggests that they may try altering typing styles or using randomized passwords.
Other potential defense measures include using software to reproduce keystroke sounds, white noise, or software-based keystroke audio filters.
Remember, the attack model proved highly effective even against a very silent keyboard, so adding sound dampeners on mechanical keyboards or switching to membrane-based keyboards is unlikely to help.
Ultimately, employing biometric authentication where feasible, and utilizing password managers to circumvent the need to input sensitive information manually, also serve as mitigating factors.
[ad_2]
Source link